Wakacoin Blockchain
Wakacoin web mining, mining while browsing the website
Sign up your Each1 account and you can mine while browsing the website.
Log in to the website and start mining by using your browser.
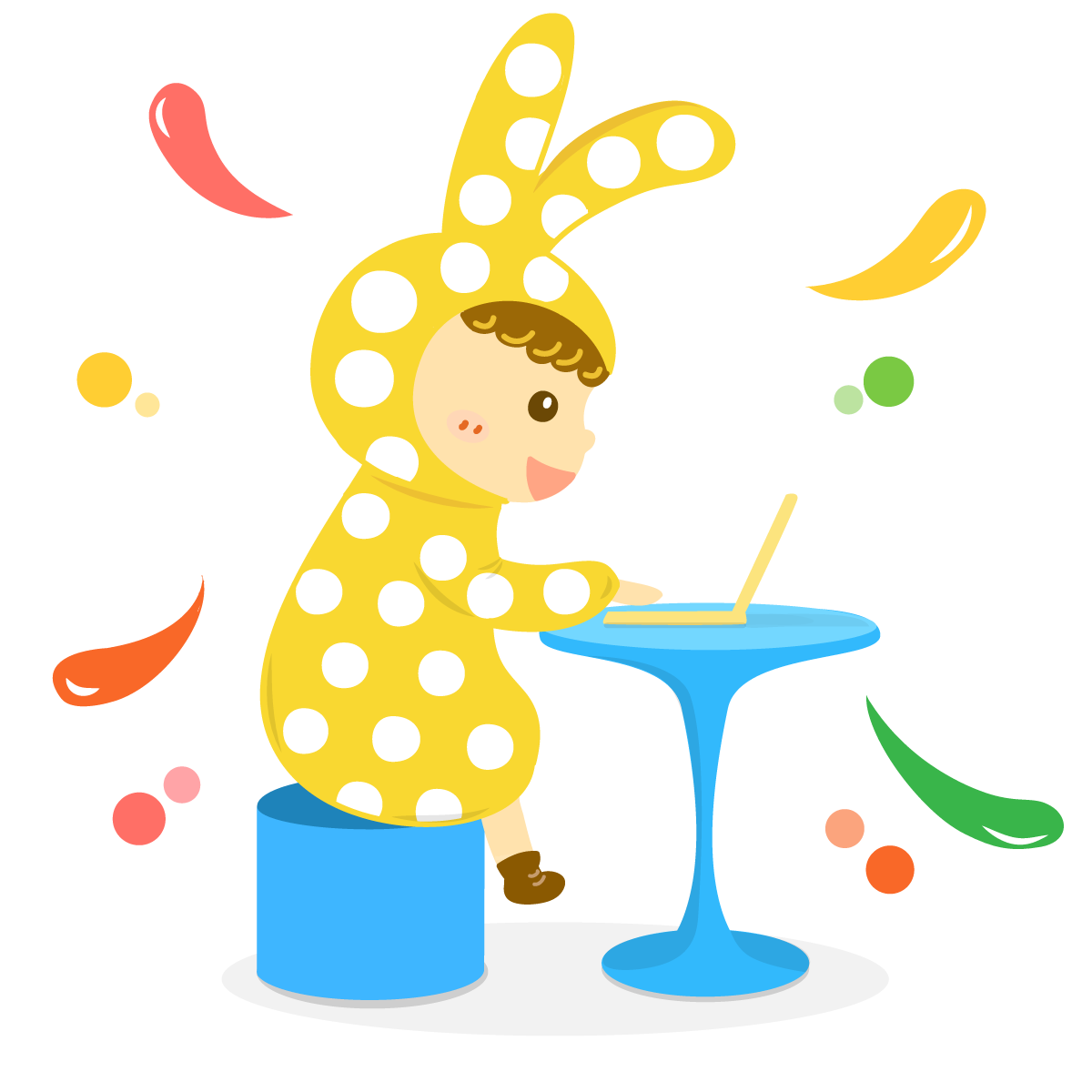
Own Wakacoin to Protect Your Assets Against Inflation
Wakacoin is a blockchain that uses PoW (Proof of Work) and generates about 50,000 wakacoins every year (one wakacoin every ten minutes on average).
For more details, please see Wakacoin white paper.
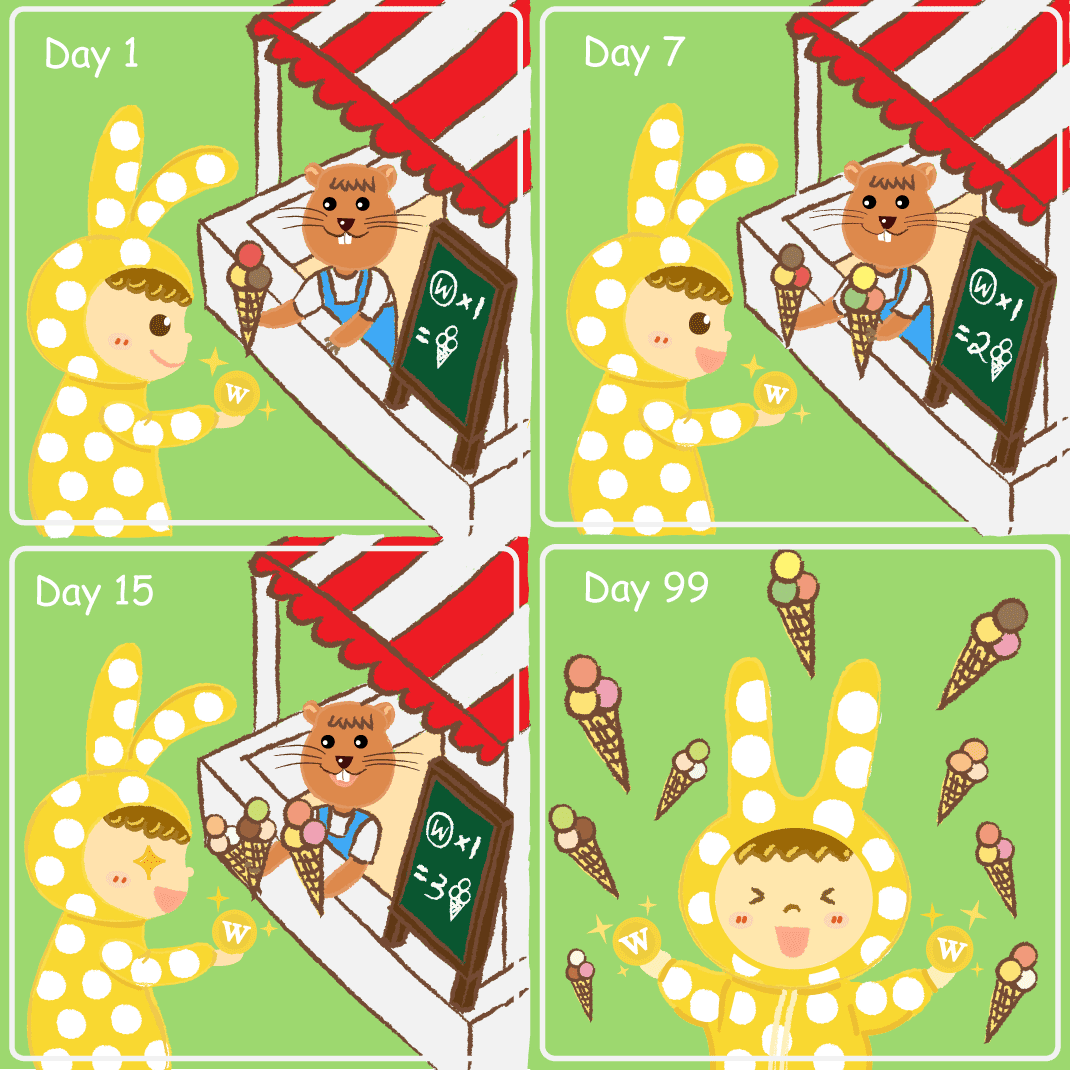
CBS (Coin Birds Stablecoin) makes digital currency easier to use
CBS is a digital currency issued by using Wakacoin Smart Contract.
According to the rules of the smart contract, the price is anchored at US$1 per 100,000 CBS.
The Coin birds help people to exchange CBS in coins, outdated currencies, or discontinued currencies.
Each1.net supports the exchange of CBS and Wakacoin forever.

Wakacoin and CBS form an infinite cycle
Wakacoin is inherently a deflationary asset.
CBS is inherently an inflationary asset.
Due to the complementary characteristics of Wakacoin and CBS, an infinite cycle is formed.
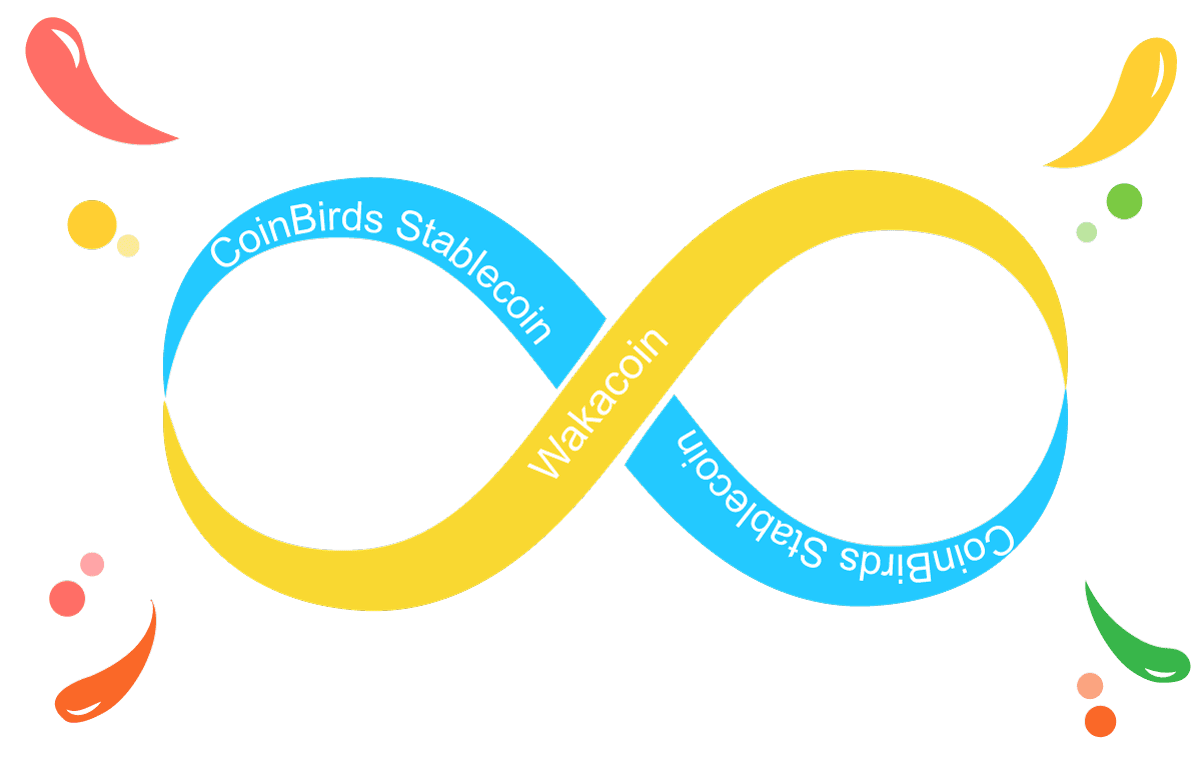